Revolutionising Healthcare with Large Language Models: Opportunities and Challenges
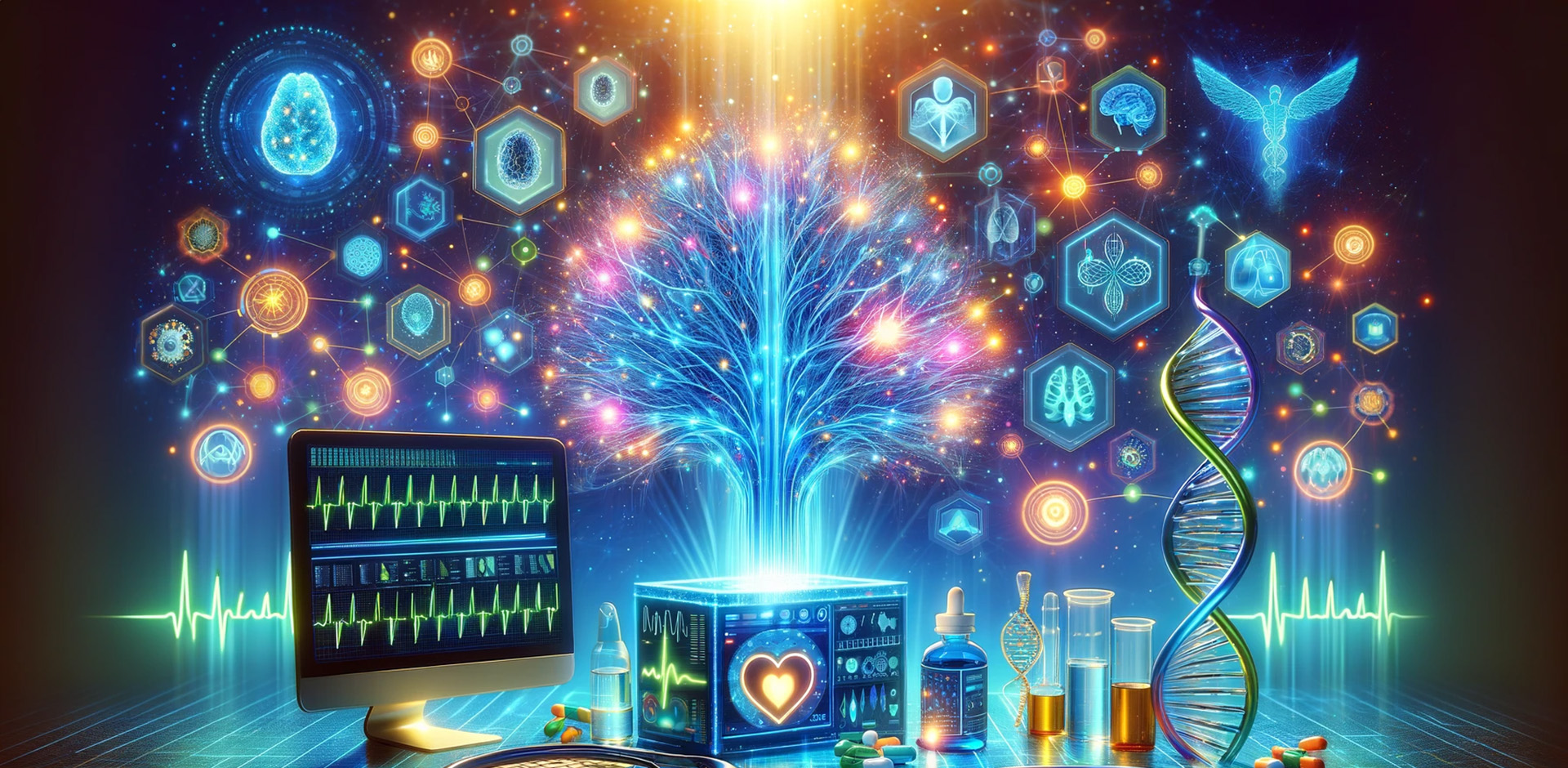
The integration of Medical Large Language Models (LLMs) into the healthcare sector marks a significant leap towards the future of medical diagnostics, patient care, and administrative efficiency. These AI-driven models, capable of understanding and generating human-like text, are transforming the landscape of healthcare by offering innovative solutions to longstanding challenges. However, the deployment of these technologies also brings forth questions regarding their accuracy, ethical implications, and the need for stringent regulatory frameworks. This article delves into the transformative role of LLMs in healthcare, explores their current applications and challenges, and outlines strategic recommendations for their future integration.
Key Highlights:
LLMs like ChatGPT and Med-PaLM are setting new standards in medical diagnostics, patient communication, and healthcare accessibility.
The potential of LLMs to democratize medical knowledge is tempered by concerns over misinformation and data privacy.
Advances in LLM technology, including multimodal capabilities, are expanding the scope of AI applications in healthcare.
Regulatory challenges loom large as healthcare systems navigate the integration of LLMs into clinical practice.
Let’s talk! Book your free strategy session now
Current State of LLMs in Healthcare
Medical LLMs, such as ChatGPT and Med-PaLM, have demonstrated remarkable capabilities in understanding and generating text based on vast amounts of medical data. These models are being utilized for a variety of purposes, including medical diagnostics, patient history summarization, and the generation of patient care plans. The ability of LLMs to process complex medical information and provide human-like interactions is enhancing the efficiency and quality of healthcare services.
Applications and Benefits
The applications of LLMs in healthcare are vast and varied. They are being used to improve patient care by providing accurate medical information, facilitating communication through language translation, and simplifying documentation tasks. Furthermore, LLMs are aiding in medical education by offering personalized learning experiences and supporting research by streamlining the analysis of scientific literature.
Challenges and Concerns
Despite their potential, the use of LLMs in healthcare is not without challenges. Concerns regarding the accuracy of the information provided by LLMs, potential biases in the models, and the risk of generating misinformation are significant. Additionally, the privacy and security of patient data when using LLMs is a critical issue that healthcare providers must address.
Ethical and Regulatory Considerations
The ethical implications of using LLMs in healthcare, such as the potential for exacerbating healthcare disparities and the need for informed consent, are areas of ongoing debate. Regulatory challenges also arise as healthcare systems and governments work to establish frameworks that ensure the safe and effective use of LLMs in clinical settings.
Future Directions and Innovations
The future of LLMs in healthcare looks promising, with ongoing advancements in AI technology. The development of multimodal LLMs, which can process and interpret multiple forms of data, including images and text, is expected to further enhance the capabilities of AI in healthcare. Additionally, the continuous improvement of LLMs through feedback loops and the integration of ethical considerations into model development are crucial for their sustainable growth.
Next Step Recommendations:
1. Develop Robust Data Privacy Frameworks: Implement stringent data protection measures to ensure the privacy and security of patient information when using LLMs.
2. Enhance Model Accuracy and Reliability: Continuously update and refine LLMs with diverse and up-to-date medical datasets to improve their accuracy and reduce biases.
3. Foster Interdisciplinary Collaboration: Encourage collaboration between AI developers, healthcare professionals, ethicists, and patients to guide the development and application of LLMs in a manner that maximizes benefits and minimizes risks.
4. Establish Clear Regulatory Guidelines: Work with regulatory bodies to define and implement clear guidelines for the development, deployment, and oversight of LLMs in healthcare.
5. Promote Ethical AI Use: Integrate ethical considerations into the development and application of LLMs, ensuring equitable access to AI-driven healthcare solutions and protecting against misuse.
6. Invest in Education and Training: Provide healthcare professionals with the necessary training to effectively utilize LLMs in their practice, ensuring they are equipped to leverage AI technologies to enhance patient care.
7. Encourage Public Engagement and Transparency: Engage with the public to build trust in AI technologies, providing transparent information about the capabilities, limitations, and ethical considerations of LLMs in healthcare.
Peyman Moh
Peyman Moh, a seasoned leader with over 20 years of experience in digital health and innovation, excels in transforming foresight into impactful realities. As the former Director of Digital Health & Innovation at GSK and founder of Foretell Innovation Lab, he has spearheaded major projects, established innovation accelerators, and provided advisory services. Renowned for his strategic foresight and ability to foster ecosystem collaborations, Peyman's expertise in future-back thinking and innovation lifecycle management positions him as a pivotal figure in navigating the rapidly evolving innovation landscape.