Revolutionising Scientific Discovery Through AI: A Comprehensive Analysis
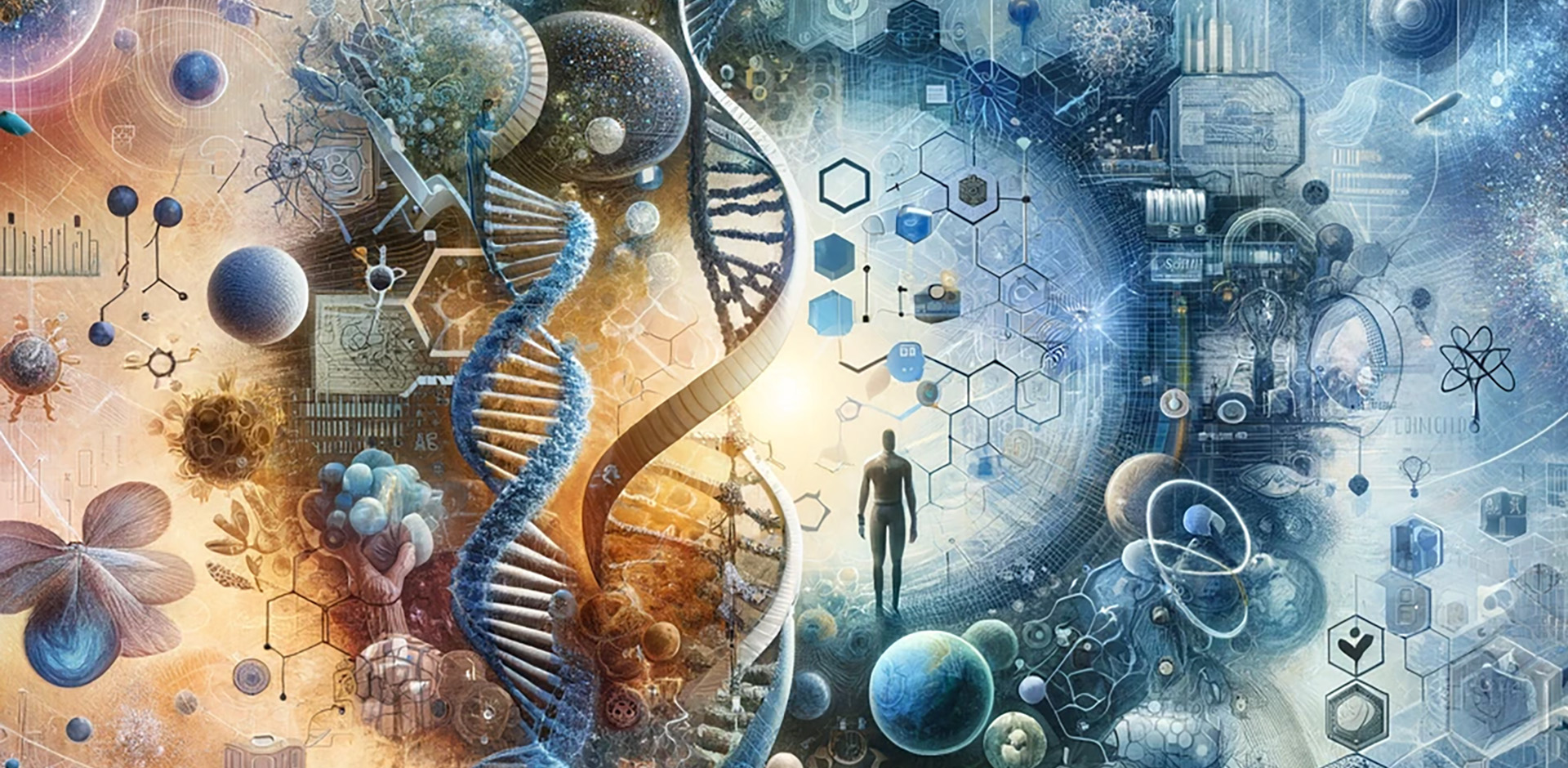
Summary
This paper explores the transformative impact of artificial intelligence (AI) and deep learning on scientific discovery, particularly within the natural sciences. As we witness an extraordinary revolution at the intersection of AI and disciplines like materials science, biology, and healthcare, it becomes evident that AI’s role extends far beyond mere data analysis. This paper delves into the challenges, strategies, and potential of AI in driving advancements in understanding and manipulating the physical world, highlighting key areas such as generative AI, diffusion models, and interdisciplinary collaboration.
Key Highlights
AI as a Catalyst for Scientific Discovery: The integration of AI and deep learning within the natural sciences accelerates the discovery and development of new materials and drugs, offering a paradigm shift in research methodologies.
Overcoming Data Challenges with AI: The scarcity and specificity of data in scientific domains pose unique challenges. AI’s ability to leverage synthetic training data and domain-specific inductive biases enables the creation of deep learning models that can accurately predict complex biological and chemical properties, overcoming the limitations of traditional research methods.
Interdisciplinary Collaboration as a Key to Success: The synergy between machine learning experts and domain specialists is crucial for the successful application of AI in scientific research. This collaboration ensures that AI models are not only technologically sophisticated but also relevant and applicable to the unique challenges of healthcare and life sciences.
AI in Life Sciences: Discoveries and Challenges
The intersection of AI and the natural sciences marks a pivotal era in human understanding and manipulation of the natural world. This paper outlines the role of AI and deep learning in enhancing scientific discovery, underscoring the necessity of specialized adaptations and the importance of interdisciplinary collaboration.
Revolutionizing Material and Drug Discovery
The application of AI in materials science and drug discovery exemplifies its potential to significantly expedite and refine the search for new compounds. Through the use of AI-driven emulators and generative models, researchers can screen millions of candidate materials or molecules rapidly, identifying promising candidates for further exploration. This process, once prohibitively time-consuming and computationally expensive, is now achievable within hours, heralding a new era of efficiency in scientific exploration.
Revolutionizing Drug Discovery with AI
The integration of artificial intelligence (AI) in drug discovery represents a ground breaking shift towards more rapid, efficient, and cost-effective processes in pharmaceutical research. AI technologies, particularly machine learning (ML) and deep learning (DL), have enabled researchers to sift through vast datasets of chemical compounds, predict their therapeutic potential, and identify promising drug candidates at an unprecedented pace.
Use case: A notable application of AI in drug discovery was seen in the development of a novel class of antibiotics. Utilizing deep learning algorithms, researchers were able to identify previously overlooked molecules within extensive chemical libraries that showed potent antibacterial activity against multi-drug-resistant bacterial strains.
Advancing Materials Science through AI
Similarly, in materials science, AI has facilitated the exploration and design of new materials with desired properties for various applications, ranging from renewable energy to biomedical devices. Generative models and AI-driven simulators have played a pivotal role in predicting material behaviours under different conditions, thereby accelerating the discovery of innovative materials.
Use case: In 2024, an AI model successfully predicted a new superconductor material capable of operating at room temperature, a long-sought goal in the field. This breakthrough was achieved by training the AI on a database of known superconductors, allowing it to extrapolate and identify potential compounds that exhibited superconductivity at higher temperatures.
Enhancing Efficiency in Scientific Exploration
The adoption of AI in these domains has drastically reduced the time and resources required for experimental trials. Traditional methods, which relied heavily on trial and error, could take years or even decades to yield significant breakthroughs. AI’s predictive capabilities, however, allow for a more targeted approach, focusing on the most promising candidates from the onset.
Use case: A cross-disciplinary team leveraged AI to streamline the development process of new photovoltaic materials in 2023. By employing machine learning models to predict the energy conversion efficiency of thousands of potential materials, the team was able to narrow down their experimental focus to the top-performing candidates, significantly speeding up the development cycle of more efficient solar cells.
These examples illustrate the transformative potential of AI in revolutionizing the fields of drug discovery and materials science. By harnessing the power of AI, researchers can navigate the complex landscape of chemical and material innovations with greater precision and speed, opening new avenues for scientific advancements and applications in healthcare, life sciences, pharmaceuticals, and medical technology.
Addressing the Challenges
Adapting AI to scientific discovery, particularly within healthcare, life sciences, pharmaceuticals, and medical technology, presents a unique set of challenges that demand innovative solutions. One of the main hurdles is the scarcity and specificity of data within these domains. Scientific discovery often relies on highly specific, sometimes sparse datasets that are not readily available in large volumes typical for training robust AI models.
Synthetic Training Data: Bridging the Gap in AI-Powered Scientific Innovation
To navigate this issue, the adoption of synthetic training data generated from simulations has proven invaluable. These simulations create realistic data scenarios that can be used to train deep learning models, or emulators, thereby overcoming the limitations posed by the availability of real-world data.
Use Case: In drug discovery, synthetic datasets generated from chemical property simulations can train AI to predict the pharmacokinetics of new compounds without the need for extensive lab testing.
AI Emulators: Accelerating Scientific Discovery with Precision
Emulators play a crucial role in revolutionizing the scientific discovery process. They are designed to mimic the behaviour of complex systems or processes, enabling rapid screening and evaluation of countless scenarios in a fraction of the time required by traditional computational methods. The speed of these AI-driven emulators does not come at the cost of accuracy. By incorporating domain-specific knowledge and data, these models can achieve high levels of precision, thereby significantly accelerating the pace of discovery without sacrificing the quality of predictions.
Use Case: In material science, AI emulators can predict the physical properties of new alloys, facilitating the search for materials with specific characteristics for use in medical devices.
Embedding Domain-Specific Inductive Biases: Enhancing AI’s Scientific Precision and Applicability
Another critical innovation in addressing these challenges is embedding domain-specific inductive biases into AI models. Inductive biases allow AI models to incorporate fundamental scientific principles and laws, ensuring that predictions not only fit the observed data but also adhere to established scientific understanding. This approach enhances the reliability and relevance of AI predictions, ensuring that the models’ outputs are feasible and applicable in real-world scenarios.
Use Case: In the development of predictive models for protein folding, incorporating biophysical principles into the AI algorithm ensures that the predicted structures are physically plausible.
By leveraging synthetic data, emulators, and domain-specific inductive biases, the adaptation of AI in fields like healthcare and pharmaceuticals is not only overcoming existing challenges but also paving the way for ground breaking discoveries that were previously considered unattainable. These advancements underscore the transformative potential of AI in accelerating scientific progress while adhering to the rigorous standards of accuracy and reliability demanded in healthcare and scientific research.
The Role of Interdisciplinary Collaboration
The integration of Artificial Intelligence (AI) into scientific research, particularly in fields such as healthcare, life science, pharmaceuticals, and medical technology, is a testament to the interdisciplinary collaboration’s pivotal role. This collaboration is not merely a beneficial addition but a fundamental component in bridging the gap between advanced AI methodologies and the intricate nuances of domain-specific challenges.
Interdisciplinary Collaboration’s Crucial Role
Interdisciplinary collaboration involves the partnership between machine learning experts and domain specialists in healthcare and life sciences. This partnership is essential for translating complex biological, medical, and pharmaceutical data into actionable AI-driven insights. For instance, in 2023, a project involving AI specialists and oncologists developed a predictive model to identify potential cancer treatments, showcasing the power of combining domain knowledge with AI capabilities.
Use Case: A notable example from 2024 is the collaboration between AI researchers and geneticists to map out gene expressions related to specific diseases more efficiently. This effort led to ground breaking advancements in personalized medicine, where AI models were trained to predict patient responses to various treatments based on genetic information.
Fostering a Common Understanding
The success of interdisciplinary teams hinges on developing a shared language and understanding. This common ground enables the effective communication of ideas, challenges, and solutions across different fields of expertise. By doing so, it ensures that AI tools developed are not only technologically advanced but also practically applicable within the specific context of healthcare and life sciences.
Use Case: In 2023, a joint effort by computer scientists and pharmaceutical researchers led to the development of an AI-driven platform for accelerating drug discovery. The platform utilized machine learning to predict the efficacy of drug compounds, significantly reducing the time and cost associated with traditional drug development processes.
Ensuring Domain Relevance and Practical Applicability
The ultimate goal of integrating AI into scientific research is to produce tools and insights that are not only innovative but also relevant and applicable to the field. Interdisciplinary collaboration ensures that technological innovations are grounded in real-world needs and can lead to practical applications, thus enhancing the impact of research on patient care and treatment outcomes.
Use Case: A 2024 initiative brought together data scientists and clinical psychologists to create an AI-assisted diagnostic tool for mental health disorders. The tool, informed by clinical expertise, improved diagnosis accuracy and personalized treatment plans, demonstrating the tangible benefits of interdisciplinary collaboration in healthcare.
Through these examples, it’s evident that the synergy between AI expertise and domain-specific knowledge in healthcare, life science, pharmaceuticals, and medical technology not only accelerates the pace of scientific discovery but also ensures that such innovations are meaningful and directly applicable to improving health outcomes.
What’s Next?
As we stand on the brink of a new era where Artificial Intelligence (AI) significantly impacts healthcare, life sciences, pharmaceuticals, and medical technology, the path forward is both exciting and laden with challenges. The advancements thus far herald the potential for AI to revolutionize our approach to scientific inquiry and problem-solving. To ensure that we harness this potential to its fullest, a concerted effort across multiple fronts is needed. The recommendations outlined below aim to provide a roadmap for future research and application in this dynamic field.
Encourage Interdisciplinary Collaborations
- The synergy between AI and domain-specific expertise has been a cornerstone of the progress made in scientific discovery. To further this, creating ecosystems that promote close collaboration between machine learning experts and domain specialists is essential.
- For instance, fostering programs that bring together clinicians, pharmacologists, and AI researchers could lead to the development of predictive models for patient response to treatment, enhancing personalized medicine strategies.
Expand and Diversify Training Datasets
- The limitation of scarce and specific data in domains like healthcare and pharmaceuticals can be mitigated by broadening the sources and types of data used for training AI models.
- For example, initiatives aimed at aggregating and anonymizing clinical trial data across multiple research centres could provide a richer dataset for AI models to learn from, potentially uncovering novel insights into drug efficacy and patient outcomes.
Focus on Domain-Specific Model Development
- Developing AI models that incorporate domain-specific inductive biases ensures that the outputs are not only accurate but also align with fundamental scientific principles. This tailored approach guarantees that AI predictions are relevant and actionable within the specific context of their application.
- For example, in the field of genomics, models that are trained to understand the complex interactions within genetic sequences can lead to breakthroughs in identifying genetic markers for diseases, thereby accelerating the development of targeted therapies.
Promote Open Access and Sharing
- The acceleration of progress in AI-driven scientific discovery is significantly enhanced when data, models, and findings are openly shared within the scientific community. This practice encourages replication, validation, and extension of research findings, driving innovation and discovery.
- For example, establishing open-access repositories for AI models trained on synthetic datasets in drug discovery allows researchers worldwide to test, improve, and apply these models to their own unique challenges, fostering a collaborative approach to overcoming healthcare challenges.
By adopting these strategies, the scientific community can continue to push the boundaries of what’s possible with AI, leading to unprecedented advancements in our understanding and manipulation of the natural world. The integration of AI into scientific research holds the promise not just for ground breaking discoveries but also for practical applications that enhance healthcare outcomes, underscoring the transformative potential of AI in shaping the future of science and medicine.
Let’s Chat! Book Your Free Strategy Session Now
Peyman Moh
Peyman Moh, a seasoned leader with over 20 years of experience in digital health and innovation, excels in transforming foresight into impactful realities. As the former Director of Digital Health & Innovation at GSK and founder of Foretell Innovation Lab, he has spearheaded major projects, established innovation accelerators, and provided advisory services. Renowned for his strategic foresight and ability to foster ecosystem collaborations, Peyman's expertise in future-back thinking and innovation lifecycle management positions him as a pivotal figure in navigating the rapidly evolving innovation landscape.